Reservoir geological control: stochastic facies modeling
- Angel Aponte
- Mar 17, 2023
- 3 min read
Updated: Apr 1, 2023
Shifting gears from MARKET BASKET ANALYSIS and RECOMMENDATION ENGINES for a little while, let's talk about Reservoir Geostatistical Modeling. A reservoir is a subsurface volume with a complex and heterogeneous internal structure. It could be described through a set of static and dynamic variables. Values for those variables can be obtained from detailed Reservoir Characterization studies: measure and interpretation of laboratory analysis of core samples; analysis of well-logs; interpretation of indirect measurements of seismic surveys; analysis of outcrops and analogs; definition of the geologic/facies models of the reservoir, etc.
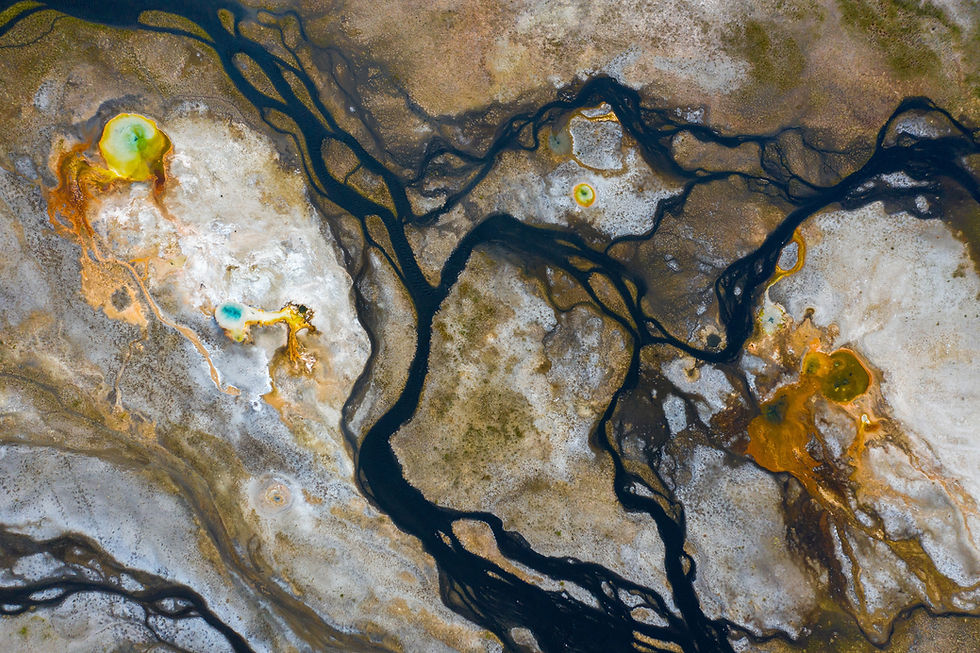
Understanding and appropriate integration of multi-scale geological heterogeneities and the SEDIMENTARY PROCESSES involved in reservoir formation are key elements for more realistic reservoir modeling, particularly for mature fields.
Regarding the construction of reservoir models, there are DETERMINISTIC and STOCHASTIC approaches. It is well known that building a stochastic/geostatistical model with solid PREDICTION POWER is a very comprehensive and complex task. So, why a deterministic approach is not enough to describe reservoir complexities and performance? Why is so important to build a reliable STOCHASTIC MODEL of the reservoir, particularly for mature fields? Key reasons could be:
Integrate the results and products of multidisciplinary studies to maximize their use and optimize the reservoir resources.
Estimate original hydrocarbon volumes.
QUANTIFY and ANALYZE uncertainty (UNCERTAINTY MANAGEMENT).
PROBABILISTICALLY, evaluate and quantify the STATIC CONNECTIVITY in the volume of the reservoir.
PROBABILISTICALLY, IDENTIFY in ADVANCE areas with potentially remaining oil (Bypassed-Oil) and locations for IN-FILL WELLS; additionally, NEW INTERVALS to be perforated, etc.
Carry out fluid-flow simulation on a set of reliable stochastically-ranked models-realizations (for example, using realizations correponding to percentiles p10, p50, and p90).
Finally, support the field development plan and the DATA-DRIVEN DECISION-MAKING process.
As mentioned above, understanding the sedimentary processes involved in reservoir formation plays a pivotal role in modeling them more realistically, allowing us to obtain solid PREDICTIVE MODELS: the ultimate goal of any modeling process is MAKING PREDICTIONS! Again, this is of paramount importance, particularly for mature fields.
The figure below shows an example that illustrates a frequent situation in which the facies model of the reservoir was not available. Along with the basic logs of a handful of wells, the volume of seismic amplitudes, and the depth interpretations of the horizons and faults, only a general conceptualization of the architecture of the sedimentary bodies was available. The model depicted was constructed in PETREL Software Platform.
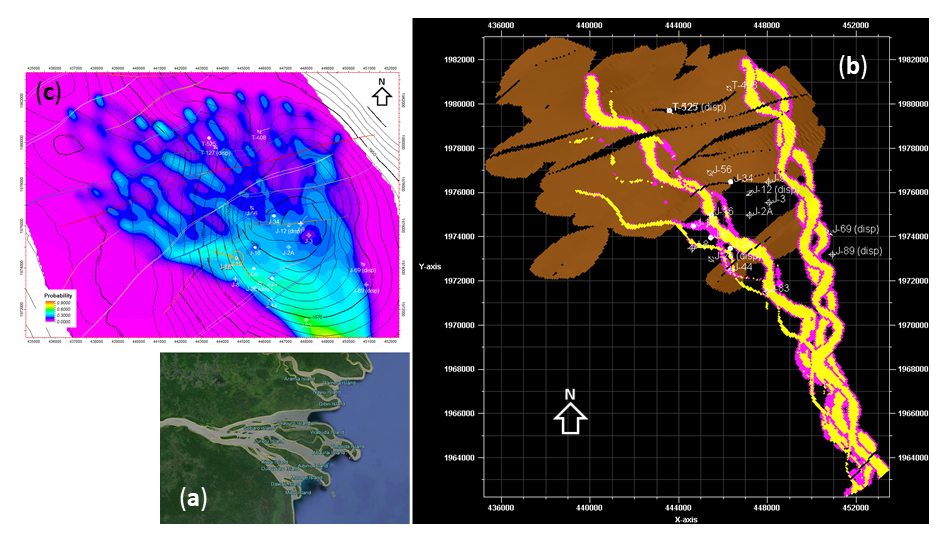
Using this data and the heuristic as a starting point, a river-dominated delta environment (a) was assumed. Then, the geometry of the main architectural elements – channel sands, levee sands, and distributary channel sands– were modeled (b).
The petrophysical interpretation of porosity, water saturation and shale volume were not available whatsoever. Despite this, using (b), it was possible to carry out a multiple realization analysis and evaluate Facies Probability Volumes (c). These probability volumes were used to identify a preliminary set of new well locations.
Indeed as this example illustrates, including at least a conceptualization of the geometry of the sedimentary bodies, substantially increases the predictive power and applicability of the resulting model; allowing for example, taking advantage of the geometric features modeled, to plan/design trajectories of new horizontal/unconventional wells, select intervals to be perforated, and estimate the volume of oil to be contacted by them. This is a clear example of how reutilizing and proper integration of even sparse datasets allows for extracting priceless knowledge, which can be used immediately to answer key business questions, particularly regarding mature fields.
Certainly, techniques and methods like those presented here could be directly adapted, as I have already done in the example presented in fifth post, to be applied in other knowledge domains: MINING INDUSTRY, PUBLIC SECURITY, RETAIL, etc.
Please leave your comments below, and kindly share and contact me if you require additional information.
Comments